Why composable architecture is the future of machine learning?
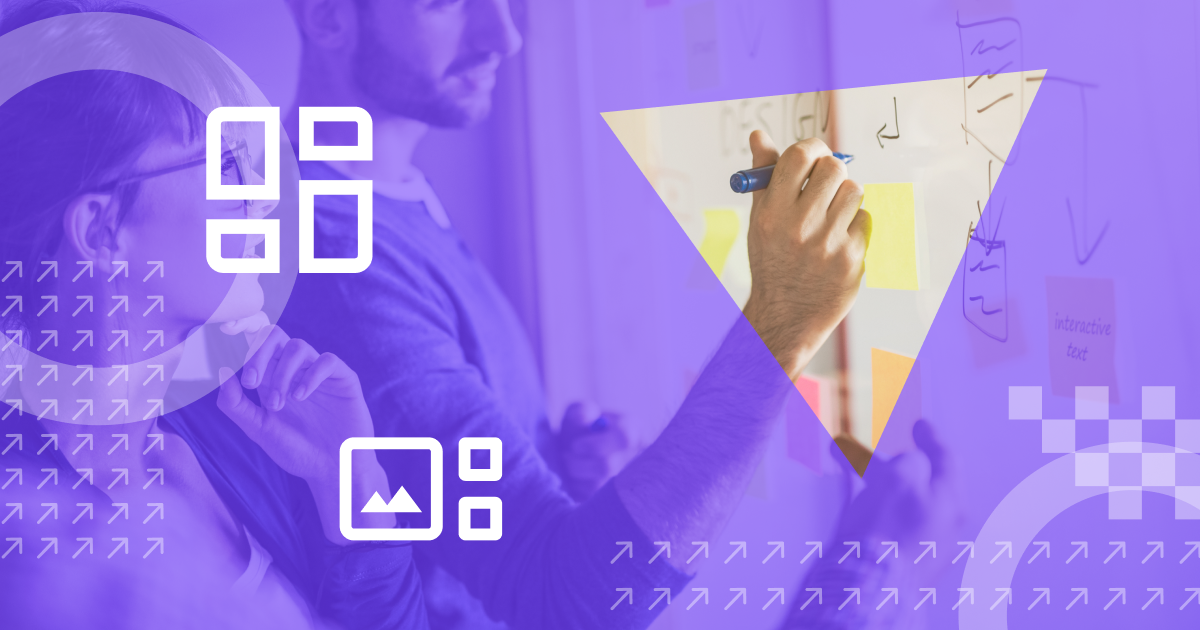
Discover how composable architecture is the future of machine learning. You can create robust, scalable, adaptable, and innovative ML models with composable architecture. Learn how Contentstack's composable architecture can keep you ahead in ML trends by scheduling a demo today with us.
Highlights
You’ll learn why composable architecture is the future of machine learning.
- Modular and reusable: Composable architecture is a new approach to developing software systems changing machine learning. It promotes the use of reusable elements, increasing efficiency and innovation levels.
- Flexible and scalable: Composable architecture can compose and decompose machine learning models. This helps you create iteration and refinement.
- Interoperable: Composable architecture is compatible with ML frameworks and tools. This ensures easy collaboration through innovation in diverse fields.
Keep reading to explore more!
Composable architecture promises businesses a lot of value as it combines the best-of-breed solutions to make your systems flexible, agile, and scalable.
According to Gartner, businesses using monolithic platforms will be 80% slower in implementing new features than competitors using modular architecture.
As a result, almost every company, whether retail, finance, e-commerce, or SaaS, prefers a composable approach. This trend is finally seeping into machine learning (ML).
In this blog, we will explore the significance of composable architecture in shaping the future of ML.
What is composable architecture?
Composable architecture is a systematic approach to building more flexible, reusable, and scalable software and systems. You create comprehensive software using independent and reusable components, breaking large and monolithic platforms into agile components. Unlike one-size-fits-all systems, a composable architecture helps you select the tools that align with your business requirements.
It includes MACH architecture (Microservices, application programming interface or API-based, cloud-native, and headless). This architecture gives businesses a high degree of flexibility and scalability and is highly extensible, allowing companies to add new features and functionalities.
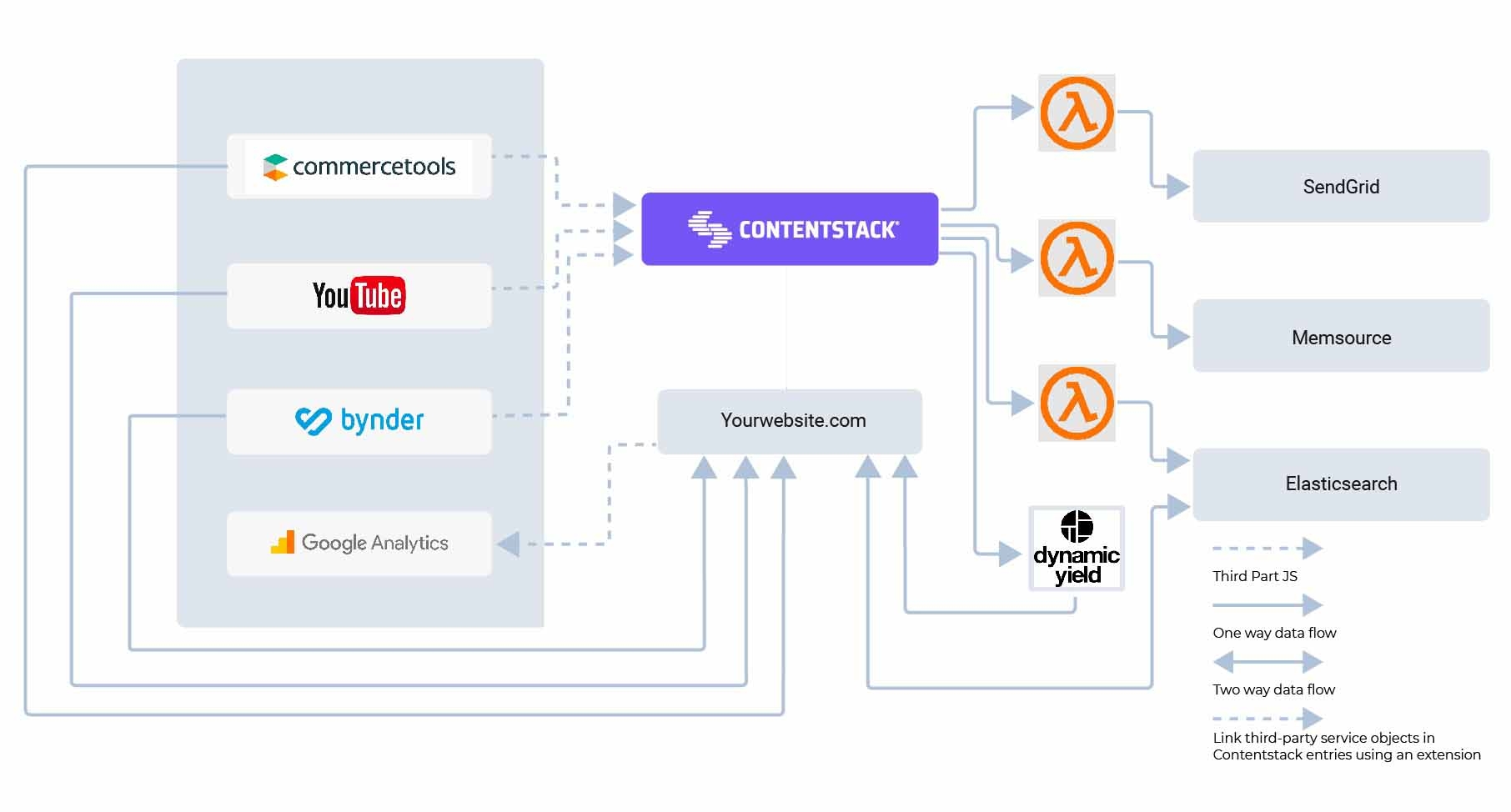
Composability in machine learning
Composable architecture pushes businesses toward an efficient and versatile tech landscape. In machine learning, it emphasizes modularity and flexibility in building systems. Instead of creating large applications, a composable approach divides AI, ML, and deep learning models into smaller, reusable components.
The technological advancement of AI and ML models is rapid, and they have varying needs, requiring an adaptable and dynamic architecture.
First, let's understand how the modular approach works for composable ML. Then, we’ll explore how ML research can assist in understanding and utilizing the composable principles.
Start your free trial with Contentstack today. Transform your brand's digital presence with Contentstack's open MACH architecture and industry-leading technology. Witness a significant reduction in publishing and development time, and elevate your content management. Start your free trial now.
Composable ML vs. ML research
Composable ML or CML is a different approach from traditional ML research. Businesses use CML to develop algorithm building blocks for models. Unlike traditional ML methods, composable ML focuses on making models and systems more scalable and flexible. This allows developers to combine various AI functionalities.
CML focuses on operations and uses a modular approach to make ML models more flexible and adaptable. For example, a business has a composable ML model for image recognition. If they want to repurpose the model, they rearrange or replace the modules to suit the new task.
This creates customized ML models tailored to specific tasks. As a result, the development and deployment process becomes faster.
Though ML research doesn’t immediately necessitate real-world applications, it focuses on future innovations in ML technology.
Composable architecture in machine learning
Composable architecture for ML is a design approach that creates scalable, adaptable, modular, and flexible ML systems and models. These ML systems help businesses achieve enhanced performance and drive innovation across various industries. Modular architecture forms the backbone for implementing composable ML.
Composable businesses can mix and match various ML components to create customized and efficient solutions. Composing and decomposing different components can create adaptable and easily scalable ML systems.
The role of composable analytics in machine learning
Composable analytics play a significant role in machine learning. It’s a type of analytics that processes a combination of different tools. Composable analytics combine data analytics services and AI into a single solution to solve a business problem. In machine learning, composable analytics can provide a way to build sophisticated data-driven models from simple building blocks.
The impact of composable analytics on business intelligence and data solutions is profound. Business users can leverage data from various sources, including social media, to make informed decisions. Composable analytics and composable architecture can lead to more robust machine learning models.
Benefits of composable architecture in machine learning
Modular architecture for ML is a boon for businesses looking to evolve and invest in modern-day ML systems. Here are some benefits businesses can reap:
Creates modular, scalable, and reusable systems
Modular architecture empowers companies to reuse pre-developed components instead of starting from the beginning each time.
This architecture approach divides large ML systems into easily manageable components. Each component has a specific role. Developers or businesses can create, test, and use them separately. Businesses can constantly fine-tune and define each component without disturbing the entire system, ensuring faster development cycles.
A pre-trained model can save time and effort in training a new one for another project. Businesses can even reuse data pipelines and adjust them for different projects, avoiding the need to create new pipelines each time.
Ensures optimum use of resources
One of the major benefits of modular architecture is its ability to facilitate efficient resource usage within companies. By strategically deploying ML components, businesses can optimize their resources.
This optimization reduces the need for extensive infrastructure, leading to significant cost savings.
For example, businesses can reuse existing components and allocate resources more judiciously instead of allocating resources to develop new ML components for every project.
Contentstack: Your partner in scaling digital experiences. Twice named a Leader in Forrester's composable DXP award, Contentstack offers an innovative approach to DXP. Experience our 100% microservices architecture and API coverage that allows instant scalability. Start your free trial today.
Promotes interoperability
Composable architectures significantly enhance interoperability among various ML frameworks and tools. A modular approach achieves this by ensuring different ML teams work together.
For instance, a team skilled in one ML framework can collaborate well with another team skilled in a different AI framework. Consequently, this cross-functional collaboration leads to the development of more holistic and robust solutions.
Let’s understand how Contentstack made LADbible Group more interoperable:
The LADbible Group team needed a CMS to handle complex content and make their systems scalable. They preferred Contentstack because of its composable architecture and extension points. This allowed LADbible to connect with existing infrastructure and try new content types.
The developer tools offered by Contentstack streamlined the development process, making it easy to roll back changes whenever required. Efficient editing ensured collaborative writing. As a result, they reduced the time spent on editing.
Contentstack made the team's experience better with good support and collaboration. LADbible quickly and cost-effectively started creating new content. They also customized 55 brand sites and improved the editing process.
After using Contentstack, Tom McGiveron, Lead Software Engineer, said,
“The APIs and SDKs [software development kits] provided make it elegant to customize and build on top of Contentstack. We use the Management API to automate the creation and configuration of stacks, and then the Extensions SDK makes it really easy to build new UI components into your content types.”
Click here for the entire study.
The future of machine learning with composable architecture
Looking ahead, composable architecture will continue to grow. Modular architecture is important because it is reusable and helps you easily make customized models.
Its flexible nature helps you experiment and iterate with various solutions, leading to breakthroughs in different fields.
But, at the same time, composable architecture has its limitations. Challenges include interoperability among the various components, dependency management, and performance optimization. Continuous research and development and adopting perfect practices are critical in overcoming these difficulties and realizing the full potential of composable architecture.
FAQs
How does composable data analytics influence the future of machine learning?
Composable analytics allows you to create adaptable and powerful machine-learning models by combining different analytical components.
What is the role of composable commerce in the digital experience?
Composable commerce allows businesses to select and assemble various commerce technologies modularly, enhancing the overall digital experience.
How does composable architecture contribute to machine learning model building?
Composable architecture allows for the rapid assembly and disassembly of machine learning models. This helps you with quick iterations and refinements.
Can you explain how artificial intelligence (AI) and composable architecture converge in e-commerce?
In e-commerce, AI and composable architecture help you create personalized customer experiences. Composable architecture allows for the flexible assembly of various AI-driven components like recommendation engines.
Learn more
Composable architecture is an exciting innovation in the field of machine learning. It offers a unique blend of flexibility, scalability, and rapid development, making it an essential tool to build complex structures easily. Are you curious about how composable architecture could revolutionize your machine-learning journey? Schedule a demo today and witness the power of this exciting innovation firsthand!
About Contentstack
The Contentstack team comprises highly skilled professionals specializing in product marketing, customer acquisition and retention, and digital marketing strategy. With extensive experience holding senior positions in notable technology companies across various sectors, they bring diverse backgrounds and deep industry knowledge to deliver impactful solutions.
Contentstack stands out in the composable DXP and Headless CMS markets with an impressive track record of 87 G2 user awards, 6 analyst recognitions, and 3 industry accolades, showcasing its robust market presence and user satisfaction.
Check out our case studies to see why industry-leading companies trust Contentstack.
Experience the power of Contentstack's award-winning platform by scheduling a demo, starting a free trial, or joining a small group demo today.
Follow Contentstack on Linkedin
Share on: